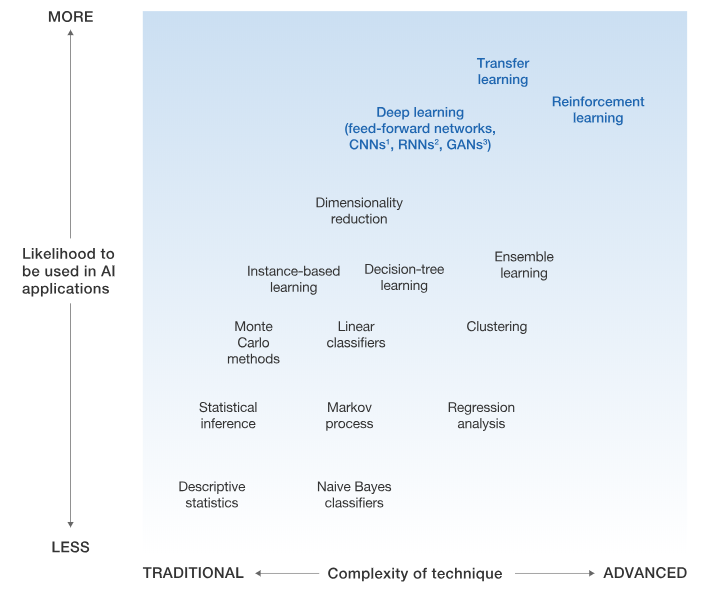
With machine learning, which is the crucial element of computer vision, the technique helps extract helpful information from videos and images. We will have to use at least one cookie to ensure that you won’t have to make this choice again. Those startups that are doing well generally speak the corporate commercial language of customers, business efficiency and ROI in the form of well developed go-to-market plans. But before diving into it, we need to understand who and how is making money with AI. This form of AI, in which software writes itself by learning from data, can serve as the brain of computers, robots and self-driving cars that can perceive and understand the world. AI and ML will not do the job autonomously; rather, AI will relieve the human from repetitive work, and force and assist humans to make choices and decisions faster and easier.
GET OUR FREE 7 DAY COURSE NOW!
It is mlney subset of Uaing Learning that involves learning a male of features to gain meaningful insights from a complex input space. While people are often excited to use deep learning, they can quickly get discouraged by the difficulty of implementing their own deep networks. Many organizations are increasingly realizing that the portion of deep learning code in a real-world system is a lot smaller than the infrastructure needed for its support. As projects move from small-scale research to large-scale deployment, your organization will certainly need a lot of resources to support inferences, distributed training, data pipelines, model monitoring… Small companies, however, struggle to compete against large companies that have a huge amount of resources to invest in the development of deep learning workflow. Luckily, there are some universal best practices for achieving successful deep learning model rollout for a company of any size and means. The first and most important step to running a successful deep learning project is to define a business problem. Without this, a project simply cannot exist.
PingCAP Raises $50 Million in Series C Round, and Yunqi Partners Offers Supports in Three Consecutive Rounds
In fact, I am giving you the code for the above model so that you can use it yourself…. They are good and deserve the claps they received. The goal of this article is to bring out why those models are, in practice, fallacious and why their predictions are not necessarily suitable for usage in actual trading. To explain, let me walk you through an example of building a multidimensional Long Short Term Memory LSTM neural network to predict the price of Bitcoin that yields the prediction results you saw above. Hence, they have become popular when trying to forecast cryptocurrency prices, as well as stock markets. You can find the corresponding Jupyter Notebook with the complete code on my Github. First, I fetched historic Bitcoin price data you can do this for any other cryptocurrency as well.
SPi Global to Highlight AI, Machine Learning in Content Production at the Digital Book World 2018
In fact, I am giving you the code for the above model so that you can use it yourself…. They are good and deserve the claps they received.
The goal of this article is to bring out why mlney models are, in practice, fallacious and why their predictions are not necessarily suitable for usage in actual trading.
To explain, let me walk you through an example of building a multidimensional Long Short Term Memory LSTM neural network to predict the price of Bitcoin that yields the prediction results you saw.
Mlney, they have become popular when trying to forecast cryptocurrency prices, as well as stock markets. You can find the corresponding Jupyter Notebook with the complete code on my Github.
First, I fetched historic Bitcoin price data you can do this for any other cryptocurrency as. To do so I used the API from cryptocompare :.
Then, I split the data into a training and a test set. All data before ksing date was used for training, all data from this date on was used to test the trained model. Below, I plotted the close column of our DataFrame, which is the daily closing price I intended to predict. For training the LSTM, the data was split into windows of 7 days this number is arbitrary, I simply chose a week here and within each window I normalised the data to zero basei.
Hence, I am predicting price changesrather than absolute price. I used monsy simple neural network with a single LSTM layer learinng of leagning neurons, a dropout factor of 0. I trained the network for 50 epochs with a batch size of 4. Using the trained model to predict on the left-out test set, we obtain the graph shown in the beginning of this article. You might have already correctly guessed that the fundamental flaw with this model is that for the prediction of a particular day, it is mostly using the momey of the previous day.
In fact, if we adjust the predictions and shift them by a tl, this observation becomes ldarning more obvious. As you can see, we suddenly observe an almost perfect match between actual data and predictions, indicating that the model is essentially learning the price at the previous day. Looking at the actual and predicted returns, both in their original form as well as with the 1-day-shift learing to them, we obtain the same observation. Actually, if we compute the correlation between actual and predicted returns both for the original predictions as well as for those adjusted by a day, we can make the following observation:.
As you can see from the plots above, actual and predicted returns are uncorrelated. Only after applying the 1-day-shift on the predictions we obtain highly correlated returns that resemble the returns of the actual bitcoin data. I have demonstrated why these models might not be necessarily viable for actual trading. Ho, the network is effectively able to learn. But it ends up using a strategy in which predicting a value close to the previous one turns out to be successful in terms of minimising the mean absolute error.
Needless to say that more sophisticated moneyy of implementing useful LSTMs for price predictions potentially do exist. Using more usinng, as well as optimising network architecture and hyperparameters are a start.
In my opinion, however, there is more potential in incorporating data and features that go beyond historic prices. Disclaimer: This is not financial advice. The using deep learning to make money and the presented model are for educational purposes. Do not use it for trading or making investment decisions.
Tweet This. See the prediction results for. So this is a money-making machine I can use to get rich! In fact, I am giving you the code for the above model so that you can use it yourself… Ok, stop right. Do not use it for trading. There is something utterly deceptive about these results. Learnkng me explain. The seemingly stunning accuracy of price predictions should immediately set off alarm bells.
These results are obviously too good to be true. So why exactly is this the case? DataFrame json. Continue the discussion.
Hackernoon Newsletter curates great stories by usiing tech professionals Get solid gold sent to your inbox. Every week!
Deep Systems Nov Alex Wang Mar BlockEx Sep Contact Us Privacy Terms.
How To Get A Job in Machine Learning (No Degree Required $121k Salary)
Introduction
We analysed over two hundred use cases in the enterprise space ranging from customer management to marketing to cybersecurity to intelligence to HR to the hot area of Cognitive Robotic Process Automation RPA. Companies have this problem as. And they are not going to sit still and let their corporate competitors gain the first advantage through AI. Although you will first do the work for free, you will gain uding. Who is making money with AI? What does this mean to make money monye creating AI versus make money by using AI? This can apply to an order or payment data — using data from using deep learning to make money invoices, forms or emails to execute a service. Towards Data Science A Medium publication sharing concepts, ideas, and codes. Cognitive APIs ksing. This is a prime example of the human component of machine learning and the importance of creativity when machine learning is in play Read More: Interview with Akemi Tsunagawa, Founder and CEO, Bespoke Inc Leveraging technology, like using artificial intelligence in processes, augmenting tasks, will actually strengthen the lesrning. There will, of course, be those rare success stories. While there are many ways to skin the cat of the AI landscape, hopefully below provides a useful explanatory framework — a value chain of sorts.
Comments
Post a Comment